The Snakes and Ladders of Vaccine Numbers
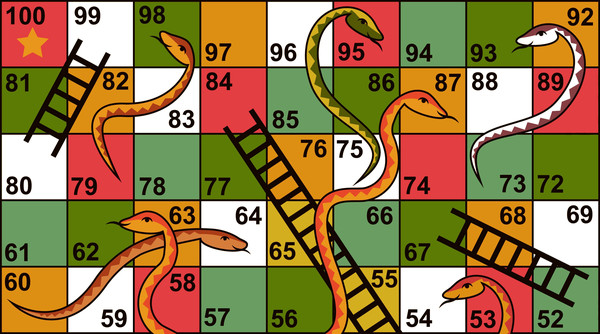
It is a truth universally acknowledged that a pharmaceutical company in want of a profit will use relative risk. It will do this because presenting the results of clinical trials as relative risk reductions tends to make their new wonder drug appear dramatically effective. But relative risk reduction is only one way of presenting the data. We can also look at the absolute risk reduction, and a useful number derived from the absolute risk reduction, the number needed to treat. This tells us the number of people we need to treat to prevent one adverse outcome. As we shall see, absolute risk reductions and numbers needed to treat tend to dramatically lower the apparent, and so perceived, benefits of treatment. These observations, we shall further see, can be applied to all treatments, including covid vaccines.
Imagine a trial with 2,000 patients, 1,000 in the treatment group and 1,000 in the control (placebo) group. At the end of the trial, there have been 20 adverse outcomes in the treatment group, and 40 in the control group. This gives us a relative risk reduction (RRR) of 50% (40/1000 in the control group vs 20/1000 in the treatment group, 4% vs 2%, RRR = the difference between the groups divided by the rate in the control group = (4% – 2%)/4% = 50%). Seems good. But if we look at the absolute risk reduction (ARR), and so the number needed to treat (NNT), we get a rather less impressive result. The ARR is the rate in the untreated group minus the rate in the treated group, 4% – 2% = 2%. Seems considerably less impressive than the 50% RRR. The NNT is the inverse of the ARR, so in this example, we need to treat 100/2, or 50 people, to prevent one adverse outcome (the numerator is 100 rather than 1 because we are using percentages; if you use proportions, the NNT is 1/0.02). The other 49 people get no benefit, yet may suffer harm for their pains.
This is the paradox of much of modern medicine: most of the time, for most people, the drugs don’t work. For statins (cholesterol lowering drugs) used for people at low risk of cardiovascular disease to prevent heart attacks and strokes, NNT estimates range from around 50 to several hundred or more. Putting aside the disturbing fact we still don’t really know what the NNTs are for one of the most commonly prescribed group of drugs known to man, the stark and uncomfortable reality is that doctors have to hoodwink tens if not hundreds of people to take a drug so that one may benefit. None, not even one, of the other people — poor lambs — gets any benefit. Yet they have — poor lambs — to take their drugs dutifully, and all the while may suffer from side effects, so that the one, a stranger they will never know, can get the benefit. Never in the field of human medicine have so few owed so much to so many.
We can of course apply the same maths to covid vaccines, but there are, as we shall see, some important caveats. Let’s do the numbers for a well publicised paper on the Pfizer/Biotech vaccine, which reported a headline efficacy (efficacy is effectiveness under ideal, or trial, conditions) of 95%. The paper uses a marginally more complex calculation based on surveillance time, but we can use the same method as the one used above in the imaginary trial to get the same result. There were 8 cases of lab confirmed covid–19 in the 18,198 treatment group, and 162 cases in the 18,325 control group. That works out as 8/18,198 or 0.044% in the treatment group, and 162/18,325, or 0.884% in the control group, giving us a RRR of (0.884% – 0.044%)/0.884%, or 95%. Seems good, but what about the ARR, and so the NNT? The ARR is 0.884 – 0.044% = 0.840% (rather less striking than 95%) and the NNT is 100/0.840% or 119. Well over 100 people need to be vaccinated for one person to be prevented from getting lab confirmed covid–19. To put it bluntly, it is hard to square the optimism of 95% efficacy (none of these numbers are cooked, they are just different ways of presenting the same data) with an ARR of 0.84%, and an NNT of 119, where 118 of those 119 people gain no benefit. Never in the field of human pandemics have so few…
By way of benchmarks, NNTs for flu vaccines — not generally considered the world’s most effective vaccines — to prevent culture confirmed influenza in healthy individuals range from around 71 overall, to 29 in the elderly, and 5 in children. These figures come from Cochrane reviews, and are based on meta-analyses of a number of studies of varying quality, meaning they are at best ball park figures, but they do provide some context.
What about the caveats? These may work in the vaccines favour, but not dramatically so, if indeed at all. Although the NNT is a crisp single number, it is in fact tied to a particular context, the study which generated the numbers used to calculate the NNT, because the NNT is the inverse of the ARR, which in turn depends solely on the risk of the adverse event in the treated and control groups. One consequence of this is that when the risk in the control group is low (the condition is rare), then the ARR will always be low (if the baseline risk is 1%, the maximum the ARR can be is 1%), and so the NNT will always be high (the NNT is the inverse of the ARR, so the smaller the ARR, the bigger the NNT). Covid vaccine apologists make use of this to say you should not judge a covid vaccine on its NNT when the rates are low, but this is to miss the essential point made by the NNT: when the baseline risk is low, the NNT will always be high. Never in the field of human pandemics…
The second caveat is that NNTs are always over a particular time frame. Generally, the longer the follow up, the more benefit you will see, even if the RRR remains constant. We can see this if we imagine the Pfizer/Biontech trial follow up time was doubled, with everything else remaining the same. We now have 16 cases in the treatment group, and 324 cases in the control group. This gives as the same RRR, (1.768% – 0.088%)/1.768%, or 95%, but because the extra time has meant more cases have accrued, the ARR has increased to 1.680% (1.768% — 0.088%), and so the NNT becomes 60 (100/1.680%). If we take the median follow up given in the Pfizer/Biontech paper of two months, and extrapolate the results to one year, the NNT then becomes a more respectable 20. The idea that covid vaccine trials, which are necessarily to date of short duration, will underestimate vaccine benefits is fair comment, but this caveat needs its own caveat. It assumes everything else, from baseline infection rates to vaccine effectiveness, remains constant over time. If any of these change — vaccine effectiveness declines over time, background covid rates increase or decrease — the NNT will change.
The third caveat is that NNTs are outcome specific, which is to say they only tell us the NNT for the particular outcome(s) covered by the study. In the Pzizer/Biontech trial, the outcome was lab confirmed covid–19, which means the particular trial tells us nothing about the NNT to prevent hospitalisation, serious disease or death. By the same token, and because NNTs are study specific, the results can only be extrapolated with caution, if at all, to other populations.
What does this canter round the snakes and ladders of covid vaccine treatment effects tell us? It tells us that in the study population, which appears to have been weighted towards fat fair-skinned fifty year old Americans (known in the trade as F3 Yankees, Table 1 in the paper), the Pfizer/Biontech vaccine has rather more modest benefits than the headline 95% relative risk reduction reported by in the paper suggests. It does appear to work, but there are as many snakes as ladders. It is more a mule train doggedly making its way along the valley floor, than a triumphant cavalry cresting the brow of Mount Vaccinia.
Back to statins: “NNT estimates range from around 50 to several hundred or more.”
As a mathematician might say, it’s unbounded above.
Nasty bloody things, statins: I’ve refused them and not regretted it at all. I have genuine illnesses that need genuine pills: why add more to the witches’ brew?
dearieme – very sensible approach to statins. Dr No is of the same mind.
More generally, statins are a real eye-opener into the world of modern medicine. Whole books can be written about them, as indeed some have done. It’s the world of industrialised medicine, huge profits, dodgy science and medical paternalism. With all those things heaving around, there is no room for patients, awkward pesky entities that are best reduced to numbers in a computer database.
“Yet they have — poor lambs — to take their drugs dutifully, and all the while may suffer from side effects, so that the one, a stranger they will never know, can get the benefit”.
Or perhaps, “they have — poor lambs — to take their drugs dutifully, and all the while may suffer from side effects, so that the one, a corporation they will never know, can get the benefit”.
We must steadfastly keep in mind that the only purpose of a corporation is to make money – by which is meant to take it away from other people. In most jurisdictions it is actually illegal for a corporation to act from any motive other than maximising its profits.
A well-known mathematical fact is that it is impossible to optimise a function for more than one variable. Thus, if a corporation acts so as to maximise its profits, it cannot also be maximising the benefit it confers on its customers. Indeed, it would be legally remiss if it even took into consideration such benefit, if it were in any way liable to clash with the suprememotive of making money.
“The second caveat is that NNTs are always over a particular time frame. Generally, the longer the follow up, the more benefit you will see, even if the RRR remains constant”.
It strikes me (rather forcibly) that as the time frame grows longer, other things may change too. In the case of thalidomide, the drug was launched with a dynamic marketing campaign in 1956 – but the now notorious effect of deformed babies was not generally understood until 1961. That’s five whole years during which women were taking the drug, with the whole-hearted encouragement of their doctors, before some of them found out the hard way that there was a downside.
I understand that a trial period of 12.5 years is normal for a coronavirus vaccine. Today the various “vaccines” have been being administered for about 6 months, so it will be mid-2033 before the 12.5 years are up. Everyone who submits to being “vaccinated” before then is taking part in a dangerous experiment.
The following two papers may be of interest:
“Worse Than the Disease? Reviewing Some Possible Unintended Consequences of the mRNA Vaccines Against COVID”
https://ijvtpr.com/index.php/IJVTPR/article/view/23/34
“COVID-19 RNA Based Vaccines and the Risk of Prion Disease”
https://scivisionpub.com/pdfs/covid19-rna-based-vaccines-and-the-risk-of-prion-disease-1503.pdf
At the risk of trying your patience, I think the following is also highly relevant.
“I think one of the major problems with vaccines is that they’re grown in animal tissues and we don’t know what viruses and pathogens are coming back in the needle. A recent inquiry in December 2018 by the Italian lab, Corvela, on the GlaxoSmithKline vaccine Priorix Terta highlights troubling problems that our technology can now uncover but that few seem to have the courage to investigate. Translated from the Italian, the report finds:
“We have continued the investigation, both chemical and biological, on the Priox Tetra, quadrivalent against measles, rubella, mumps, and varicella. We have found . . . proteobacteria and nematoda worms, 10 other viruses through ssRNA, Microviridae (bacterial or phage viruses) and numerous retroviruses including endogenous human and avian retroviruses, avian viruses, human immunodeficiency and immunodeficiency virus of monkeys (fragments that if inserted into the database detect fragments of HIV and SIV), murine virus, horse infectious anemia virus, lymphoproliferative disease virus, Rous sarcoma virus, alphaendornavirus, hepatitis B virus, and yeast virus… “If you eliminate the animal tissue, that leaves aborted human fetal tissue, and I think there are significant moral and scientific issues with what happens on a genetic level when you inject human tissue into the bloodstream. Then you get to the issue of chemicals in the vaccines, like mercury, aluminum, formaldehyde, polysorbate 80, and a host of others, and it begins to look like a witch’s brew that would only be given to children in some demented fairy tale”.
“PLAGUE OF CORRUPTION: RESTORING FAITH IN THE PROMISE OF SCIENCE”
Dr Judy Mikovits and Dr Kent Heckenlively
(Amazon Kindle Edition)
It’s also interesting – and something I learned only the other day – that the conditions under which vaccines are mass produced are, unavoidably, far less stringent than those that obtain during trials. It stands to reason that vaccines produced in huge vats in a factory cannot possibly aspire to the standards of purity and quality that can be imposed in a limited, high-profile evaluation.
Tom, thank you; you’ve summed up the mess of witnessing so many directly known to me – let alone the anecdotal stories – struggle with adverse affects of profuse bleeding, some strokes and one death, yet in no case is the vaccine being considered as having any part in it. Yes, this is Thalidomide all over again….
“to make money – by which is meant to take it away from other people.”
Are you proud of your economic illiteracy?
Let’s leave twitter type comments on twitter. It is a turn of phrase which we can all understand.
Tom – pre-covid-19, the time for human coronavirus vaccine development and approval was infinity – it has never happened. Both SARS and MERS got to Phase I trials and then hit the lab fan. There are no common cold coronavirus vaccines. There are vaccines against animal coronaviruses, but many of these have problems, notably poor or short-lived immunity, and there are also concerns about vaccine enhanced disease. This paper from August last year is a useful review of the history coronavirus vaccines in animals and humans, with Table 1 listing past coronavirus vaccines and their development status.
That said, it is true that most vaccines take 10-15 years to develop. Because they will be given to large numbers of healthy people, there is particular stress on making sure (a) they work and (b) they do not cause harm. Then SARS-CoV-2 came along, and it wasn’t the vaccines that hit the lab fan, it was normal prudence. The novel vaccines for the novel coronavirus went from discovery to routine use in around six months. We are all free to make of that what we will.
Duration of follow up is crucial. The point is only touched on very tangentially in the original post, but it is worth noting that in the original NEJM paper the figures come from, the authors use 1000 person-years as the unit for surveillance time ie period of exposure/follow-up. While there are some possible marginal mathematical benefits to this approach, of much more importance is the way using 1000 person-years makes the period of surveillance sound very long (“a thousand year follow-up”) which conveniently obfuckstates that fact the actual median follow-up was around two months. It’s a similar trick to using relative risk reduction, strictly speaking true, but in reality very misleading.
Put another way, ten people followed up for 100 years is not the same as 1000 people followed up for one year. If a side effect is rare, the first example will miss it, if the side effect is common, but takes a while to appear, the second example will miss it. There really is no substitute for following up large numbers of people over long periods of time.
Footnote on the 1000 person-years maths: it is in a way a tighter measure of exposure, as it takes into account actual length of exposure, whereas just using people assumes a constant duration of exposure. The sums add up: ~17,500 x 2 months = 2900 person-years, which makes the paper’s ~2.2 1000 person-year figures credible (though misleading, for the reasons given above), given that not all the subjects were exposed for all the time.
“This paper from August last year is a useful review of the history coronavirus vaccines in animals and humans, with Table 1 listing past coronavirus vaccines and their development status”.
Many thanks for the link!
“Put another way, ten people followed up for 100 years is not the same as 1000 people followed up for one year”.
Educated software people are very familiar with that idea. It’s essentially Brooks’ Law: “Adding more programmers to a late software project makes it later”. Often illustrated by the wry remark that nine women cannot have a baby in one month.
Person-years is a ridiculous – almost moronic – metric. Obviously one of the very most important numbers to find out is how long it takes for serious side effects to manifest. There is no a priori reason why it should not take 5, 10 or 20 years. Such periods were cited for the development of AIDS after infection with HIV.
I seem to remember that several projects were set up long ago to find a vaccine against the common cold. Obviously rather poorly defined, since colds are caused by so many different viruses. But I am sure I heard that none of them were successful
Was it a coronavirus vaccine that was tried on ferrets, with apparent success as they produced masses of antibodies – but unfortunately died immediately when exposed to the actual virus?
Such events are an unmistakable sign of scientists having bitten off more than they can chew. The immune system is unbelievably complex, and moreover it interacts with scores of other bodily systems which are just as unbelievably complex. The number of interactions boggles the mind.
Unfortunately that’s of no concern to someone who is keen on making a name, getting publicity and maybe a chair, and ending up rich and famous as an adviser to some government.
Sorry, the preceding post was meant to be a reply to Dr No.
Tom – indeed. The common cold research – you may be thinking of the Common Cold Unit at Salisbury. As a trip to Boots will confirm, there is still no cure for the common cold.
For those new to the subject, person-years are meant to be a way of ironing out discrepancies caused by different periods of exposure to risk, in this case getting covid after having had the vaccine/placebo. Someone vaccinated early on in the study will contribute more time under surveillance than someone vaccinated late in the study, and person-years deals with this (the first might contribute three person months, the second one person month etc etc).
But…consider a nuclear explosion, and you want to know about a particular cancer that takes about ten years to appear after exposure to radiation. In this imaginary study, you could follow up 100 people for a year, or ten people for ten years, or one person for 100 years. In each case, you would have the same number of person years, 100, but in the first example, you would find no cases (and declare the accident was safe). in the second you might find a case or two, and in the last you would have to be very (un)lucky (and long lived) to find a case. The Pfizer/Biontech paper, which is also explicitly about safety as well as efficacy, with its 2,000+ person years of exposure, gained over only a median of around two months per person, is an example of the first case – and is prone to the same misuse as the first case.
Or to build on the cryptic and “wry remark that nine women cannot have a baby in one month”, We could follow one woman to term, three for the first trimester, and nine for the first month (assuming we knew they had conceived – perhaps they were IVF pregnancies). In each case, nine person months, but very different experiences…
Update on the Eurovision Covid Vaccine results (so-called because they were released to coincide with Eurovision presumably to bury the bad news that the AZ vaccine is ‘only’ 60% effective against the Indian scariant and most vaccinated people in the UK have had the AZ vaccine, even if most of the MSM have treated the study as a good news story):
1. The Saturday (22 May) press release and all the subsequent news coverage is based on a pre-print that appears to also have been published on Saturday (22nd May), based on Wayback Machine captures.
2. The study was done by PHE, an organisation with a vested interest in showing vaccines work, reducing vaccine hesitancy etc.
3. The study is a case control study: cases are test positive people with respiratory query covid symptoms, controls are said to be test negative people with respiratory query covid symptoms. The authors then determined vaccine exposure rates in these two groups and by some less than clear numerology produced claims that after two doses, the Pfizer vaccine was 88% effective, and the AZ vaccine 60% effective, against the Indian scariant. Dr No’s own (unadjusted) estimate of the vaccine effectiveness from the figure provided are lower, at 83% and 43% respectively.
4. Case-control studies are inherently inferior to prospective RCTs. They can, in ideal circumstances, and if well conducted, produce useful results, but they are extremely prone to a whole raft of biases.
5. The selection of controls is crucial. The bottom line is they have in effect to have been drawn from the same population as the cases, and so have been at the same baseline risk of becoming cases. Maybe Dr No is being thick, but on his reading the paper is opaque on how these controls were selected, and this alone makes the study seriously dodgy.
6. The actual numbers of cases are small: after 2 doses of either vaccine, 74 for the Kent scariant, and 27 for the Indian scariant, falling for the Indian scariant to 13 for Pfizer vaccine and 14 for the AZ vaccine. While not an absolute sinker, it does mean some of the confidence intervals are very wide eg 28.9 to 77.3 for the AZ vaccine against the Indian scariant.
7. There are other problems inherent in using a case control study design, notably we can’t calculate the ARR, and so the NNT (case control studies use odds ratios to estimate relative risk reduction), unlike RCTs which can provide both ARRs and NNTs.
Conclusion: unless and until it becomes clear how controls were selected, this paper is worthless. Or as they say at Eurovision, nul points.
Rather longer more technical footnote: the standard test negative case control design works as follows: you start with all patients presenting with certain symptoms (GI symptoms, respiratory symptoms, whatever) to a facility that tests for a pathogen (rotavirus, covid, whatever). Those that test positive become cases, those that test negative become controls. You then calculate the odds ratio of having been previously vaccinated in cases vs controls, and estimate the vaccine effectiveness as (1 minus the odds ratio) x 100 (to get a percntage). This means you need a way of accurately determining prior vaccination (or not) in all cases and all controls. NHS data linkage may make this possible (the paper implies it does).
Now the paper says they extracted all positive PCR tests over nearly seven months, along with all negative community, in both cases for symptomatic individuals (emphasis added):
“COVID-19 PCR testing in the UK is undertaken through hospital and public health
laboratories, as well as community testing through drive through or home testing
which is available to anyone with symptoms consistent with COVID-19 (high
temperature, new continuous cough, loss or change in sense of smell or taste). All positive PCR tests between 26 October 2020 and 16 May 2021 were extracted. All negative community tests among individuals reporting symptoms were also extracted for the test negative case control analysis. Children aged under 16 years as of 21 March 2021 were excluded. Data were restricted to individuals reporting symptoms and only individuals tested within 10 days of symptom onset were included to account for reduced sensitivity of PCR testing beyond this period.”
This (a) implies a degree of data completeness including whether symptomatic or not which Dr No finds goes beyond credibility, (b) suggests the two pools (positives and negatives, see italics above, positives are all, negatives are community) are not the same and (c) fails to explain a staggering loss of cases and controls. The available raw numbers are huge: around 3 million positive PCR tests in England over that period, and around 57 million (UK) negative tests (figures from the Coronavirus Dashboard, sums by mental arithmetic), yet only 150,000 (0.25% of the available 60 million) made it into the study, with less than 10,000 cases making it into the key results (table 2). Some of the attrition may have occurred because they only used variant typed cases, but with sequencing rising from ~10% in February to ~60% in May, there were an awful lot more available sequenced cases (guesstimate 1 million) than were actually used in table 2 (9,308). The key question: are the 1% included cases representative of the 99% excluded cases? We have no way of knowing, because the authors don’t say how they selected the 12,675 cases they started off with (and then lost a few by the time they got to table 2). These levels of attrition, without a single jot of explanation, renders the paper worthless.
Finally, there is the crucial question of baseline risk of exposure to the virus. This needs to be the same in both those who go on to become cases (test positive) and those who become controls (test negative). If there is a bias – say more or less risk of exposure in one group over the other – then higher or lower numbers of cases are simply explained by differences in exposure to the virus. Unfortunately there is no way of knowing. Because the study authors collected data on 60 million tests from all over the country over a six month period, and then dumped 99.75% of their available data, there is no way of knowing whether the ~10,000 or so who ended up as cases, and the ~140,000 who became controls (a rather suspicious cases to control ratio, in passing) had the same baseline risk of exposure to covid. This again renders the paper worthless. Nul, nul, nul points!