More Trouble with Antibody Tests
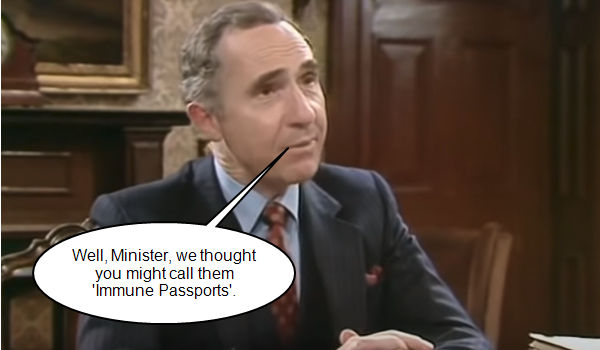
As an appendix to yesterdays post, Dr No is going to do a run through of another reason why high hopes of antibody testing being a game changer will most likely turn out to be false hopes. It is also an introduction or aide-mémoire to the more general question of how do you go about establishing the usefulness of a diagnostic or screening test, using screening for covid-19 antibodies as an example. As always, complex formulae and other numerological devices will be avoided in favour of plain words and simple numbers. You really can do these sums on the back of an envelope.
The starting point is that you have a condition – in this case, prior infection (which might still be current, if in the later stages) with covid-19, and a gold standard test which establishes the existence of the condition, in this case the PCR test that tests for the virus.
The first assessment of the new test is to see how good it is at finding the condition when it is present, and not finding the condition when it is not present. These two measures are known not unreasonably as sensitivity (a sensitive test picks up more positives) and specificity (a specific test isn’t too troubled with false negatives). If we use the familiar two by two table with disease yes/no across the top and test positive/negative down the side we might have something like this:
Disease present | Disease absent | Total | |
Test positive | 90 | 10 | 100 |
Test negative | 10 | 90 | 100 |
Total | 100 | 100 | 200 |
We can now calculate how many with the disease tested positive as a percentage of all those with the disease (90/100) and how many of those without the disease tested negative (90/100), and say that the test has a sensitivity of 90 %, and (as it happens) a specificity of 90%.
Now, the trouble is, sensitivity and specificity (the figures most often made available) are of very little to no real world use. They do matter as a general screening test of the test — a test with low sensitivity and/or specificity is a non-starter — but even when the two are reasonably high, as in the example given here, that tells us very little about real world usefulness.
The reason is that sensitivity and specificity don’t answer real world questions, instead they are merely characteristics of the test. To calculate either or both, we need to know how many people definitely, by the gold standard test, have the condition, in which case we don’t need to do the test, since we already know who does and doesn’t have the disease. It is an example of an exact answer to the wrong question (how good is the test when we already know the answer), an inverse of the real questions we want to answer, which are firstly how many people with a positive test have the disease, and how many people with a negative test don’t have the disease?
At this point traditional texts and regrettably too many modern ones with take you on a diversion through the back roads of Bayes theorem and likelihood ratios, where you encounter an increasingly baffling terminology and formulas drawn by spiders who have first travelled across the ink pad. This is by and large a plot by mathematicians and statisticians to confuse ordinary lay and medical folk, and can safely be left on one side.
Instead, what we want to know are two measures, one known as the positive predictive value (PPV, what percentage of people with a positive test actually have the disease) and negative predictive value (NPV, how many people with a negative test don’t have the disease). In covid-19 antibody testing, the PPV is particularly important. We shall see why in a moment.
We can apparently calculate the generalised PPV and NPV easily enough from the table. From the above table, of the 100 who tested positive, 90 had the disease, so the PPV is 90%. Likewise 90 of the 100 negatives didn’t have the disease so the NPV is 90%.
But in fact we have done a bit of numerology, in effect a sort of reverse engineering of the sensitivity and specificity in a given set of conditions, in particular a fixed prevalence (prevalence is the percentage of people with the disease, in this case 50%).
But what happens if we have a different prevalence while keeping the same sensitivity and specificity?
Now, this is the point where we need our thinking caps on. Let us say the disease has a much lower real world prevalence than it has in our sensitivity and specificity study. This means that even a low false positive rate can quickly build up the absolute number of positive tests (positives, but many are false positives), so diluting the PPV. The general rule, is that for a given sensitivity and specificity, the lower the prevalence in the real world compared to that in the sensitivity/specificity test, the more the PPV is diluted.
So we need a way to incorporate this effect into our calculation of the PPV (and NPV) in the real world. For this we need to know, or at least estimate (because we don’t yet know it in the case of covid-19) the real world prevalence. Let us say it is 5%. We already know the prevalence in the sensitivity and specificity study was 50%, and that is not an unusual prevalence in such studies — the investigators take so many people with disease, and a similar number without the disease.
Now let us redo the table, adjusting the prevalence to 5%, and keeping the sensitivity and specificity at 90%. What we get is this:
Prevalence now set to 5% | Disease present | Disease absent | Total |
Test positive | 9 | 19 | 28 |
Test negative | 1 | 171 | 172 |
Total | 10 | 190 | 200 |
The sensitivity, the number of people with the disease who tested positive, is still 90% (9/10 = 90%) and the specificity, the number without who tested negative, is still 90% (171/190 = 90%). But the PPV has taken a huge hammering. The false positives now outweigh the true positives (19 to 9) and the PPV, the percentage of true positives to all positives, has plummeted from 90% to 32% (9/28 = 32%). By the same token the NPV (true negatives/all negatives) has gone up (171/172 = 99%).
Now, in the real world, sensitivities and specificities of around 90% will often be considered ‘acceptable’. But if they are based on artificially high prevalence rates (as they often are, an ‘accident’ of the experimental design), then in the real world, where prevalence may be much lower (say 5%), then the PPV starts looking rather feeble.
If the above hypothetical scenario (50% prevalence in sensitivity/specificity testing, 5% real world prevalence, neither being that unreasonable) is applied to covid-19 antibody testing, then out of every three positives, there is one true positive (has had the infection) and two false positives (have never had the infection), but yet, under the tomfoolery currently doing the rounds, all three will get ‘immune passports’.